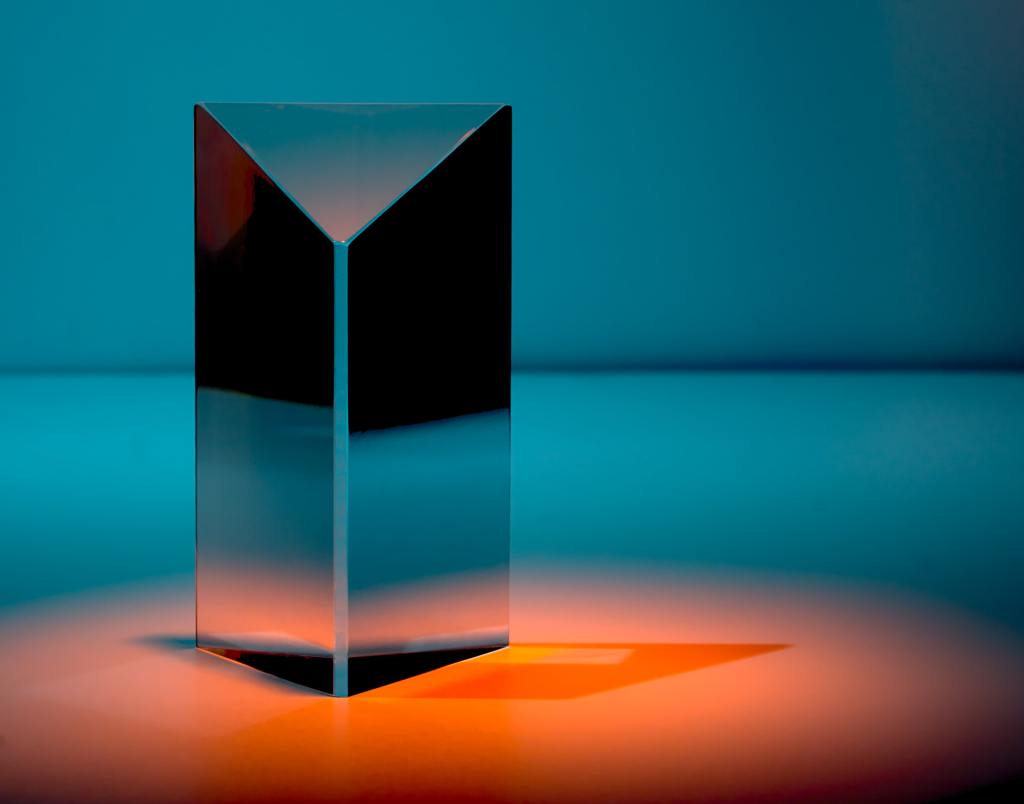
Artificial Intelligence(AI) holds a lot of potential to most industries but has not caught on yet. It has transformed the way big consumer companies like Google, Baidu, Amazon work. These companies have the benefit of massive data collected over many years from millions of consumers.
However, companies in manufacturing, agriculture, healthcare, in particular, need to find a way to make AI work for them. These companies would require a large number of customised solutions for their use cases.
They need to think of a different approach. They should build AI systems with data that helps AI to learn what it should facilitate. The data used should convey information on the important cases and is classified consistently so that AI can learn from them. Hence the IT teams should learn to program with data instead of programming with code.
Adopting AI can be difficult.
The main reasons why AI has hardly been used outside internet companies are as follows:
- Small datasets: In consumer companies, it is easy to collect large amounts of data from their consumers. In other industries, the datasets are much smaller. Ai requires large amounts of data to learn and identify the solutions. This is not possible or very difficult in other industries.
- Cost of customisation: Consumer companies employ an army of skilled engineers to build and enhance AI systems that have the potential to create tremendous value for the organisation. This luxury is not available for companies in other industries. moreover, the demand for AI talent drives up the costs.
- The gap between proof and production: Even if Ai is successful in test environments, it requires massive engineering effort to deploy in real situations.
For Ai to realise its full potential there is a need for a systematic approach to solving problems across industries. A data-centric approach is one of the ways to make this a reality.
Data-centric AI development.
Ai systems are bundles with software as well as the data used to train the AI model. An engineer may develop an Ai model to solve a production issue and then the data is fed into the AI software to help it learn from them.
During the last decade, a lot of attention has been invested in model-based development where the data is static and teams strive to optimise or invent new possibilities with the data.
the current roadblock is to get the proper type of data the Ai program can learn from. It is more beneficial to have good data rather than bug data to help Ai programs learn. The data should be comprehensive and cover all the important issues and be consistently tagged or named.
Shifting the focus from software to data offers other benefits. It relies on existing data as well as existing manpower. It allows experienced manpower in the field to contribute to developing the AI system.
Make building an AI system systematic and repeatable.
The focus on the data-centric approach is facilitated by special tools that make building, developing AI systems much easier. These tools aid in producing high-quality datasets which would help address the issues of smaller datasets, the high cost of customisation and getting AI onto the production floor.
The high-quality datasets help AI learn from smaller datasets that are available. It uses domain experts instead of AI engineers to conclude, and thus make Ai more widely accessible across industries. The tools provide the skeleton software to take the AI system into production quicker.
There is plenty of scope for AI systems in other industries. Some important things companies can do:
- Consider the quality of data collected instead of the quantity of data.
- Ensure the team takes a data-centric approach and not a software centred approach.
- Plan the de[ployment process and use the special tools to enable it.
The greatest untapped opportunity for AI is in other industries with limited datasets. This requires a change in the approach as to how we develop and deploy AI systems. The recommendations discussed above would ensure that the development and deployment are done quickly using the existing resources and that the benefits of AI are available to all.
AI Doesn’t Have to Be Too Complicated or Expensive for Your Business
by Andrew Ng HBR 2021/07